Would you prefer to work with automation that solves problems just like yourself? Would such individual-sensitive automation be able to overcome the acceptance issues observed with the introduction of new decision-support automation? This PhD research aims to find out.
In the Air Traffic Management domain, automation is expected to enable the necessary traffic capacity that is needed to meet the future demand for air travel. However, introduction of increasingly sophisticated automation is at risk of being rejected, and threatening the required growth. This article discusses ongoing PhD research that is exploring the benefits of individual-sensitive automation capable to solve conflicts between aircraft as intelligently as an air traffic controller.
Exploring automation bias
We have reached a crossroads in automation evolution where everyday technology is maturing from replacing physical tasks to assuming more and more of the “thinking” cognitive tasks previously performed only by humans. Given current development of machines and what they are capable of, it seems possible that one day machines will match and even excel human intelligence. The ultimate test for artificial intelligence (AI) was posed famously roughly sixty years ago by English mathematician Alan Turing: a computer can be said to really “think” when one can converse with an unseen agent and perceive that agent as human when in fact it is a computer. According to several leading scientists, achieving true AI (also known as point of singularity or transcendence) is not a desirable step in evolution, drawing up a dark and doomsday-like future where machines takes over the world and decides to eradicate the inferior and error-prone human race. Perhaps luckily then, to date, not a single computer has passed the Turing test and we have not realized in any meaningful way the highest levels of autonomous systems. Others are less pessimistic, foreseeing a world where human and machines live and work together and live happily ever after. Looking beyond the philosophical and moral concerns of AI, the possibility of automation as intelligent as a human being poses an interesting thought: WHAT IF we could create automation that thinks and solves problems exactly like a human, would the human more readily accept its advice? Or would the human reject the proposed solutions simply because it came from a machine? In other words, is there a dispositional bias against working with automation?
The MUFASA research
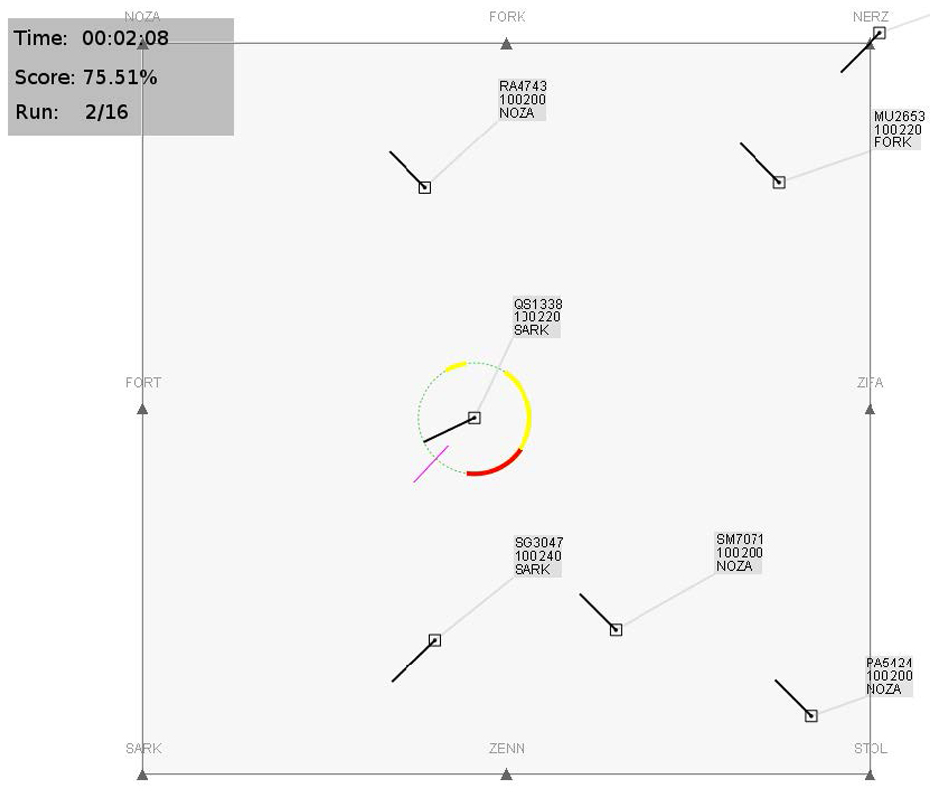
In essence, these questions drove the Multi-dimensional Framework for Advanced SESAR Automation (MUFASA) project that started in 2011 and subsequently laid the groundwork for an ongoing PhD research initiative. The MUFASA project explored these high-level questions in the context of Air Traffic Management (ATM). The ATM domain is an example of a high-risk, stochastic, dynamic, and complex environment where human and machine work closely together to achieve a common goal. The demand for air travel is pushing the envelope for what the current ATM system is capable of handling without applying restrictions on the flow of traffic. Predictions of future growth in the skies, shows that fundamental enhancements and changes are needed to modernize the close to saturated ATM system. One of the key areas in this process is the increased dependence on automation. Not only is automation expected to perform more of the routine air traffic controller tasks, but increasingly oversee aspects of more strategic and cognitive tasks, such as conflict detection and resolution. Progressively, automation is primed to mature into a team member and colleague rather than a device or tool. However, evidence from a variety of fields, including ATM, has highlighted operator acceptance as potentially one of the greatest obstacles to successfully introducing new automation. Without acceptance, the potential safety and performance benefits of automation may not be achieved. One growing line of thought is that differences between human and machine in their problem solving styles creates a mismatch that threatens the acceptance and use of sophisticated decision support automation. Researchers have addressed this issue by studying the cognitive qualities of air traffic controllers, extracting their heuristic decision-making processes, and attempting to model and package it as automation. While results have been promising, showing benefits to acceptance and automation use (for example Kirwan & Flynn, 2002; Lehman et al., 2010; Flicker & Fricke, 2005), these studies have not been able to ensure complete harmony between human and machine decision-making styles. In these studies, the human operators have been “chunked” together and treated as a homogenous single decision-making entity. Consequently, the resultant automation becomes a “one size fits all” creation that forces adaptation from the human operator. Note that this assumption is true for the majority of technology applications. In contrast, is it not likely that human cognitive characteristics in decision-making vary individually just like human physical characteristics? It seems reasonable to assume that automation thinking and solving problems similarly to the human operator would benefit teamwork and team performance.
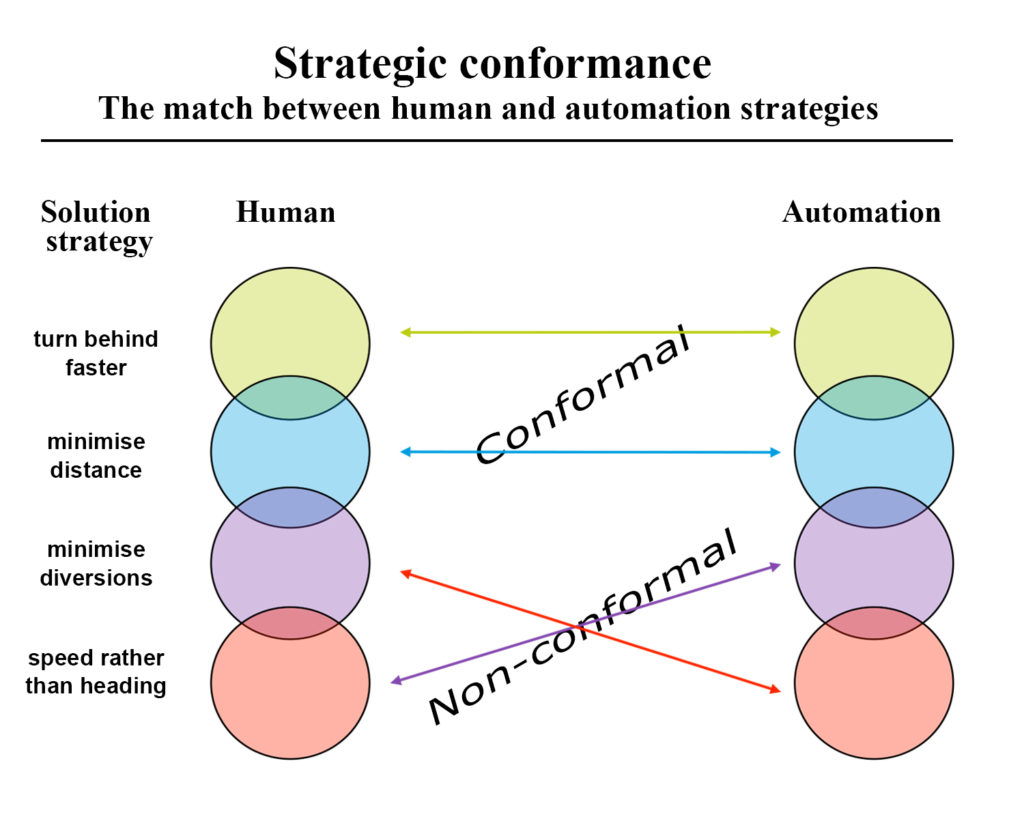
The core task in ATM consists of safely directing aircraft from origin A to destination B without colliding with other aircraft. In the tactical sense, this is represented by the conflict detection and resolution task carried out by air traffic controllers, whereby potential conflicts between two or more aircraft are identified and solved. In this specific context, the main research question of MUFASA was formulated: would controllers more readily accept advisories on how to solve conflicts between aircraft from an automated agent if those advisories were identical with how they themselves would solve the conflict? The measure of similarity in problem solving is defined as strategic conformance. This question is supposed to provide two important answers: the degree to which humans have a bias against automation, and if there are any benefits to individualizing automation.
Results indicate that strategic conformal automation can benefit automation acceptance
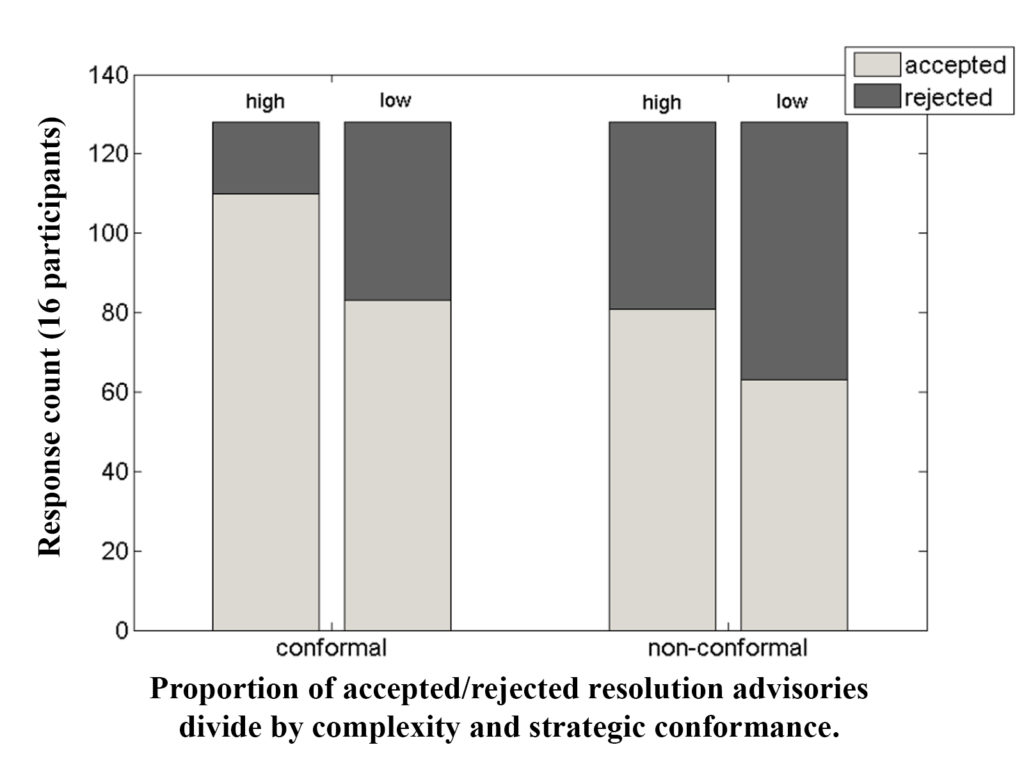
Until now, the MUFASA project has, through a series of experimental human-in-the-lop simulations involving real air traffic controllers, produced some fascinating results in addressing this question. Instead of attempting (and certainly fail) to develop full AI to assure an underlying match in intelligence between automation and human, the MUFASA team developed a decision-support system that used conflict resolution performance from individual air traffic controllers to reverse-engineer decision advisories on how to solve conflicts. Analysis of controllers’ conflict solution performance revealed large differences in conflict resolution strategies between controllers (Figure 1). In the largest trial, 16 Irish en-route controllers played a number of traffic scenarios supported by a decision support system that would suggest solutions to detected conflicts between aircraft. Controllers could, in real time, either accept or reject the suggested solution. In half the cases, the solutions were a replay of their own solution to the same conflict, as recorded in an earlier simulation. In the other half, the solution was derived from a colleague (who had chosen a different but workable solution). Data was analyzed to determine the influence of whether automation had matched controllers’ own strategy on factors such as acceptance, agreement, workload, and response time.
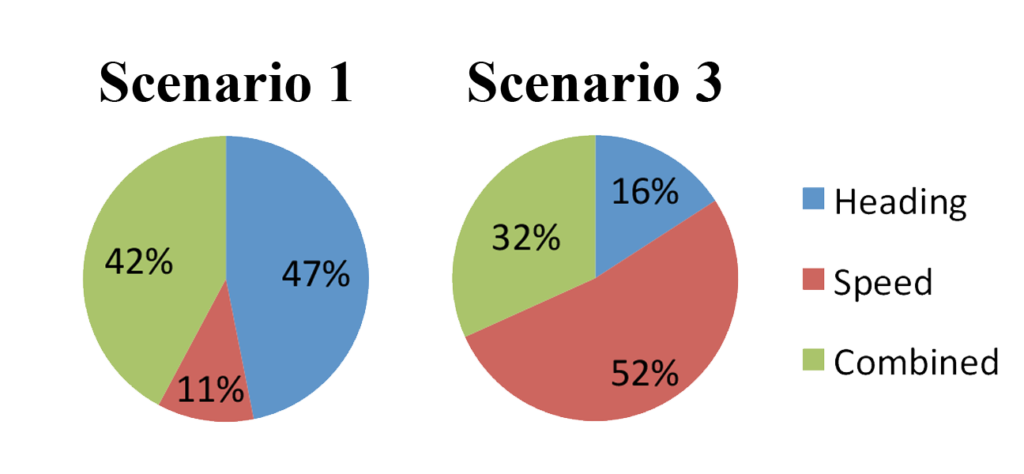
Not only did controllers accept and agree more with solutions that matched their own previous performance; they also responded faster to these solutions. These findings suggest that individual-sensitive automation can greatly improve automation acceptance and use. It underlines the important role that strategic conformance can have on the willingness to embrace new decision support automation, and that such automation should be further evaluated.
The presence of a dispositional automation bias has thus far not been established but the noteworthy result in which controllers’ rejected their own previous solution in almost 25% of the cases (believing that it came from automation) warrants further inquiry into this matter. Would controllers have been more or less accepting if they had believed the source of the advice was from a colleague? Although possibly the high count of rejections is not at all indicative of a bias but can simply be attributed to inconsistencies in human problem solving and decision-making behavior. These and other remaining questions are currently being explored in an ongoing PhD research initiative.
Disclaimer: The initial MUFASA project was a SESAR WP-E funded collaboration between Lockheed Martin UK, the Technical University of Delft (TUD), the Center for Human Performance Research (CHPR), and the Irish Aviation Authority (IAA).
[1] Brynjolfsson, E., & McAfee, A. (2014). “The second machine age: Work, progress, and prosperity in a time of brilliant technologies”, New York, NY: W. W. Norton & Company Inc.
[2] Kirwan, B and Flynn, M. “Investigating air traffic controller conflict resolution strategies”, EUROCONTROL, Brussels, Belgium, Rep.ASA.01.CORA.2.DEL04-B.RS, Mar. 2002.
[3] Lehman S, Bolland S, Remington R, Humphreys M. S, and Neal A. “Using A* graph traversal to model conflict resolution in air traffic control”, in Proc. 10th ICCM, D. D. Salvucci and G. Gunzelmann, Eds., Philadelphia, PA, Aug. 5-8 2010, pp. 139–144.
[4] Flicker R, and Fricke M. “Improvement on the acceptance of a conflict resolution system by air traffic controllers”, Baltimore, MD, Jun. 27-30 2005